“Digital twins” simulate spread of COVID-19 on Toronto public transport
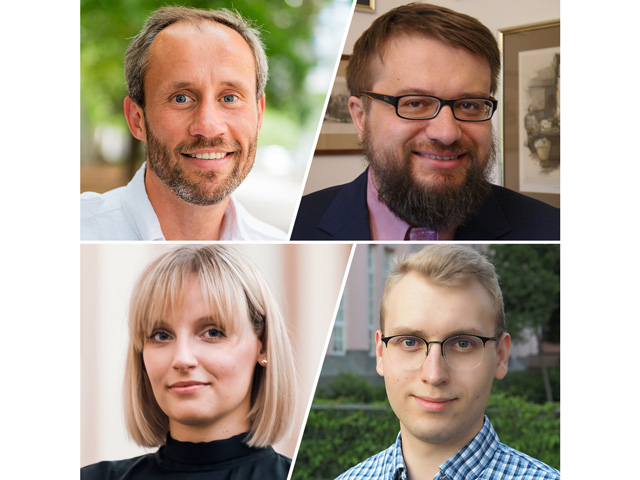
Resarch team, including professors Pawel Pralat, Przemyslaw Szufel, and PhD students Kinga Siuta, Nykyta Polituchyi
Rapidly changing data, impromptu lockdowns, to mask or not to mask — these and myriad other variables have kept policymakers scrambling to manage infection rates throughout the COVID-19 pandemic. At the core of the problem: lack of predictability.
It’s a confounding challenge — one that, in time past, was largely impossible to penetrate. But with the tremendous power of mathematics and computerized simulations, new predictive tools are making it increasingly feasible for key decision makers to peer into the potential future — and test out “what if” scenarios of all kinds. One of the latest in the toolkit is a “digital twin” that simulates the correlation between the spread of a virus, such as COVID-19, and Toronto's public transit system (TTC).
With almost 19 times* greater chance of contracting the virus indoors than outdoors, how frequently should subways and streetcars run in order to minimize risk to commuters? As often as possible? That might seem intuitive. But after thousands of simulations, the new digital twin found the optimal sweet spot: every nine minutes. The results illustrate the power of high-quality simulators to uncover the nonobvious — and to potentially help avert costly, even deadly, errors in high-risk, high-stakes contexts.
The research was developed at Ryerson University by professor Pawel Pralat of the Department of Mathematics, Przemysław Szufel, an adjunct professor of the Cybersecurity Research Lab at Ted Rogers School of Management and professor at the Warsaw School of Economics, in partnership with Toronto-based cybersecurity firm Security Compass (external link) .
Toronto’s New “Digital Twin”
Whereas some simulation models lack the accuracy of 1:1 correspondencies, digital twins — or simulating agent-based models (ABM), as they’re more formally known — are real-time, digital counterparts of an actual physical object, in this case, the city of Toronto.
“It’s essentially a virtual city, all based on actual geographic data of commuter routes and demographic data for the Greater Toronto Area. It’s as close to reality as you can get,” says Pralat. “We can replicate what’s happening in Toronto, but inside a computer. We can also play around with different scenarios without changing anything in the real Toronto — all for free and at no risk to people.”
Inside this virtual Toronto, the research team investigated infection rates based on various conditions and data — from subway and streetcar frequencies and delays, to changing commuter decisions, such as use of shortcuts or alternate routes, or even weather conditions that keep commuters underground on rainy days or out on the streets on sunny days. The potential exists to further test variables such as the percentage of people wearing masks, the impact of stay-at-home orders, and more.
Notably, the research team used emerging programming language, Julia, to code their model. "The project has demonstrated the usability and the power of the Julia language for constructing 1:1 life-scale simulation models of virtual cities,” says Szufel. “This approach allowed us to model and understand the dynamics of pandemics in a real-world city and its public transportation system."
Flexible Framework, Potential Beyond COVID
A key outcome of the research was determining the optimal subway or streetcar frequency to limit viral spread. The answer was not intuitive, but it results in the best proportion of commuters spreading out between outdoor routes and indoor transportation. The finding demonstrates the advantage of computer-assisted decision making, particularly where solutions are not immediately obvious.
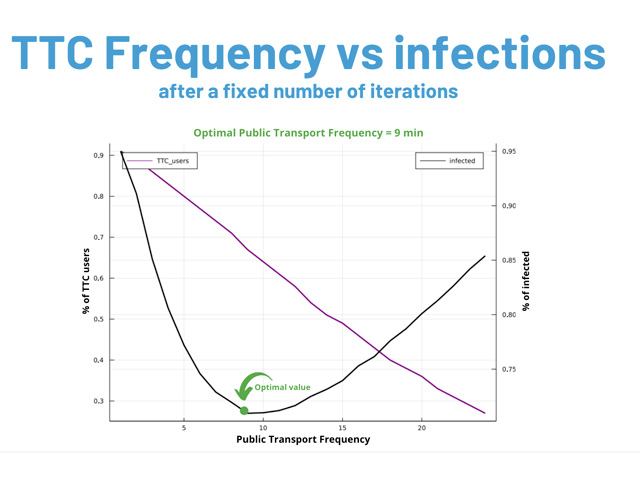
Simulations revealed that while TTC riders decreased as waiting time between subways/streetcars increased, percentage of passengers infected decreased initially, but then rose again. To minimize infections, ideal TTC frequency was nine minutes.
Altaz Valani, Director, Insights Research at Security Compass is now talking with Toronto Municipal members on the possibility of using the model in practice. He says: “This research effort is in line with other government digital transformation efforts aimed at using digital twins to simulate various municipal scenarios where a live experiment is simply not possible.”
The firm is also using the simulation to derive a proposed set of privacy guidelines aimed at developers of contact tracing apps. “This type of digital modeling can also be extended to a business context, namely, to better understand the privacy impact of contact tracing apps,” says Altaz. “For example, if only a few people are in a particular vicinity where contact tracing is permitted, it may be much easier to identify a single individual.”
Beyond pandemics, the digital twin could also be used in many other future contexts — from where to place hospitals to best service a population, to where to build roads to reduce traffic congestion, and more.
“The tools we’re building are universal and very powerful,” says Pralat. “We can ask any question without changing anything in the real world. Many questions have yet to be asked. Our model could help predict the optimal solution. Sky’s the limit.”
*According to a study (external link) conducted by University of California
The full project team included: Professors Pawel Pralat and Przemysław Szufel, PhD students Kinga Siutta, Nykyta Polituchyi and Calum MacRury, and Altaz Valani, Erik Service and Mina Miri of Security Compass. Funding was provided by the Natural Sciences and Engineering Research Council of Canada (NSERC) Alliance program, complemented with the Southern Ontario Smart Computing Innovation Platform (SOSCIP) (external link) COVID-19 Response Program.