Predicting type 2 diabetes with machine learning
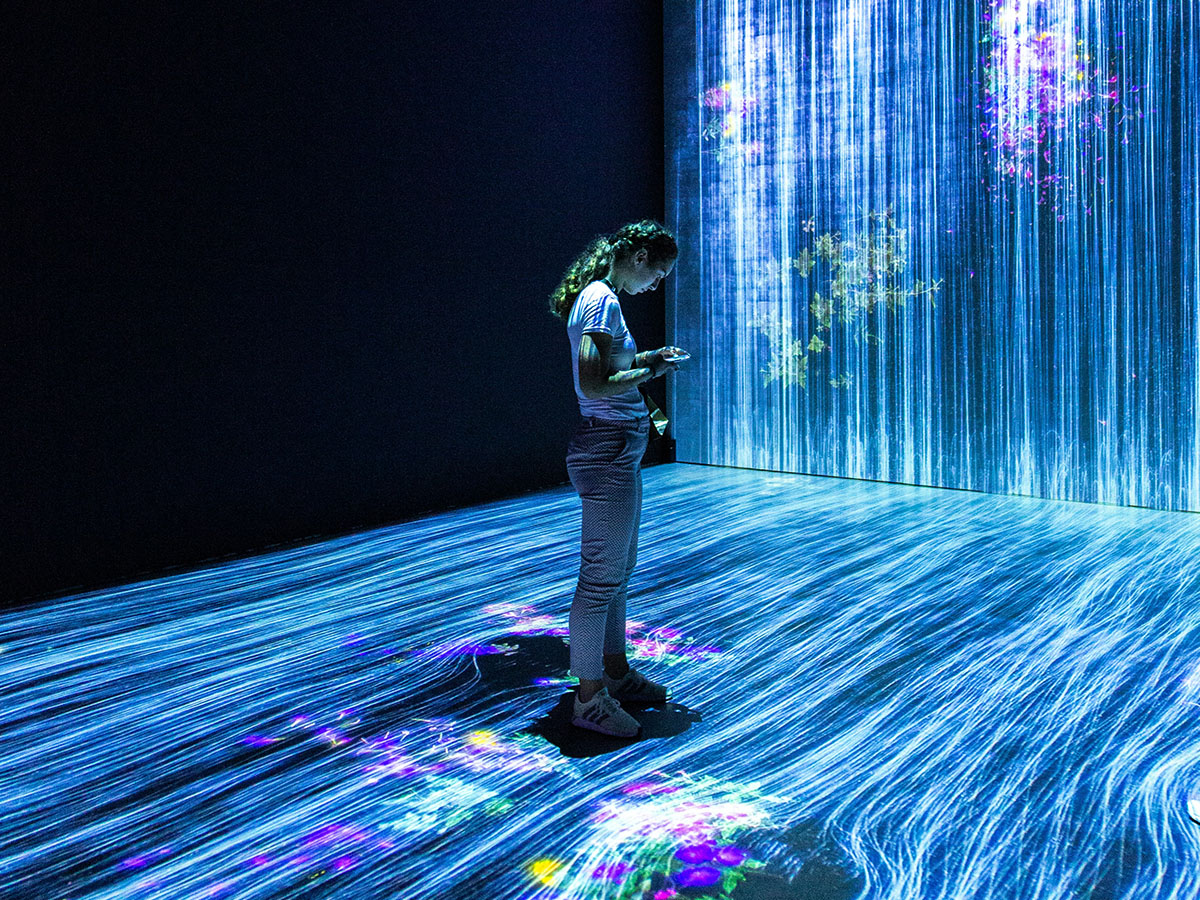
In recent decades, the number of people with type 2 diabetes has risen dramatically around the world, leading the World Health Organization to classify it as an epidemic. As with other chronic diseases, the condition is among the leading causes of deaths globally and places significant burdens on those suffering, their caregivers and health-care budgets.
Professor Aziz Guergachi of the Ted Rogers School of Information Technology Management is researching new methods to help doctors predict the likelihood of patients developing type 2 diabetes. To do this, he and his fellow researchers have created machine-learning models that analyze medical records and identify the individuals who are most at risk.
According to professor Guergachi, such innovations are urgently needed to stop people from developing preventable diseases like type 2 diabetes in the first place. By spotting the warning signs earlier, people can be encouraged to make lifestyle changes that avert the onset of the disease.
“How can we stay healthy without it necessarily costing too much?” he said. “We think that the only viable solution to fixing this health-care system is prevention.”
With health-care systems worldwide under strain from dealing with people who are already sick, professor Guergachi says there are fewer resources available for disease prevention. And while methods do exist for predicting diabetes, his Research Lab for Advanced System Modelling is finding ways to improve them.
“We’d like to change the current system by using predictive analytics,” he said. “When you look at the data, you do see that type 2 diabetes is a lot more predictable than other diseases or health problems.”
In a recent study published in the Nature Research journal Scientific Reports, professor Guergachi and his co-researchers found that their new method was more accurate at predicting diabetes up to eight years in advance than the widely used Framingham Diabetes Risk Scoring Model (FDRSM). The researchers applied a machine-learning technique known as a Hidden Markov Model to analyze a large dataset of electronic medical records, which contained key information about patients’ health over time. Risk factors for type 2 diabetes included blood pressure, body mass index, cholesterol and blood glucose levels.
The model developed by professor Guergachi and his team had the advantage of taking into account measurements from multiple points in time, allowing it to predict progression towards diabetes more successfully than the FDRSM, and to better identify low-, moderate- or high-risk patients. Overall, the Ryerson model had an accuracy of 86.9 per cent, compared with 78.6 per cent for the FDRSM.
“In our paper, we showed that the model we proposed improves the accuracy of type 2 diabetes prediction, which will help make preventative interventions more cost-effective,” said professor Guergachi.
While prediction models for type 2 diabetes are already in use, professor Guergachi argues they are not readily available to doctors. He contends the ultimate goal should be to develop an automated system that analyzes medical records and instantly provides health-care professionals with risk scores for their patients.
“We always keep thinking in terms of automation, but you would have to be careful and think about the risks,” he said. “You would need very sophisticated processes for that automation.”
Professor Guergachi is now planning to work on a pilot with doctors in Toronto to do further experiments with the model. Yet to support doctors in the long term, he maintains that a new type of prevention organization may need to be established to proactively contact patients when they are flagged as “at-risk.”
“We need to build some processes to help doctors deal with those high-risk patients,” he said.
With new forms of health-care data becoming available in the digital age, including biometrics from smart wristbands, professor Guergachi is confident that his lab can continue to support the enhancement of predictive methods for chronic diseases.
This research was partially supported by the Natural Sciences and Engineering Research Council of Canada (NSERC).