Modelling and Mining Complex Networks as Hypergraphs
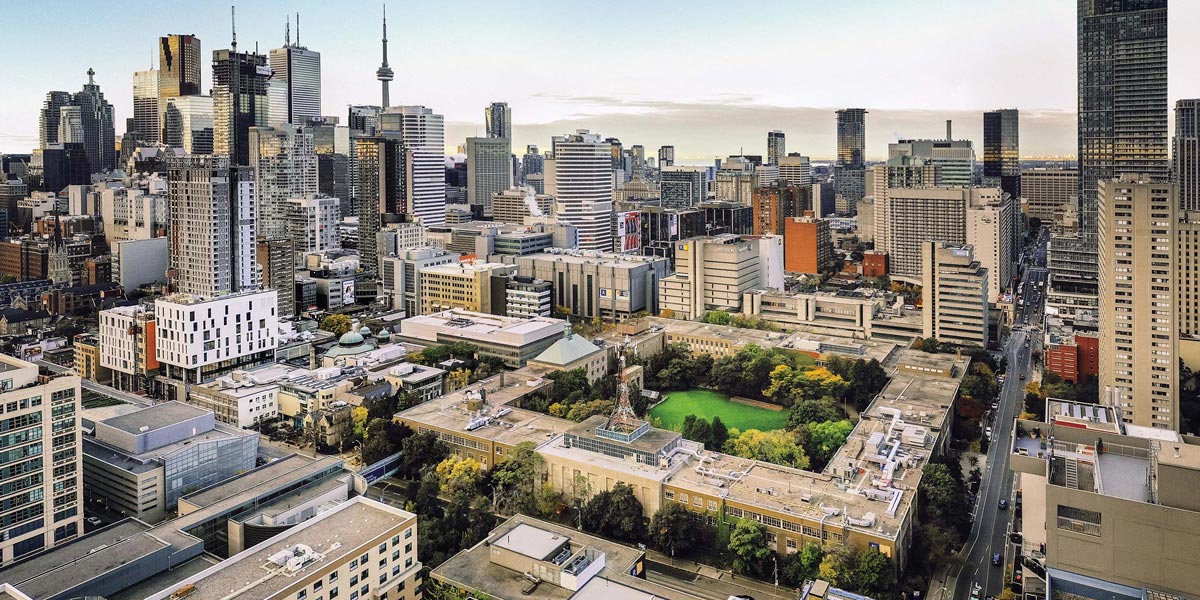
Date: May 6-9, 2024 (Monday - Thursday)
Host: Toronto Metropolitan University (opens in new window) , Toronto, ON, Canada
Location: AOB-1270 (Atrium on Bay). Please refer to Campus Maps.
Address: 20 Dundas Street West (external link) . If you enter the building from Yonge Street, you can take the elevators between Muji and OLG. We're on the 12th floor, FOS entrance.
Registration: If you are interested in this event, please send an e-mail to pralat@torontomu.ca
Organizers:
- Bogumil Kaminski, SGH Warsaw School of Economics, Warsaw, Poland
- Pawel Pralat, Toronto Metropolitan University, Toronto, Canada
- François Théberge, Tutte Institute for Mathematics and Computing (TIMC), Ottawa, Canada
Hypergraphs have recently emerged as a useful representation for complex networks since they allow capturing more general relations than graphs. Research on the generalization of various graph-based tools and techniques is booming and, in parallel, new software packages are being developed. Having said that, the theory and tools are still not sufficiently developed to allow most problems to be tackled directly within this context.
The main goal of the 4 day workshop is to gather together a small group of experts who specialize on modelling and mining complex networks represented as higher-order structures such as hypergraphs. Long talks (2 x 75 minutes) in the morning will aim to introduce specific problems in this context and explain solutions as well as tools used to solve them. Shorter talks (30 minutes) in the early afternoon will concentrate on open problems and unsolved questions to stimulate collaboration and discussions in the late afternoons and evenings.
There is no registration fee but please let one of us know that you plan to attend (to make sure we have enough space and food). The workshop will include theoreticians interested in developing theory of hypergraphs, practitioners interested in building ML tools for hypergraphs, and economists interested in incorporating such solutions in real-world business applications.
Invited Speakers
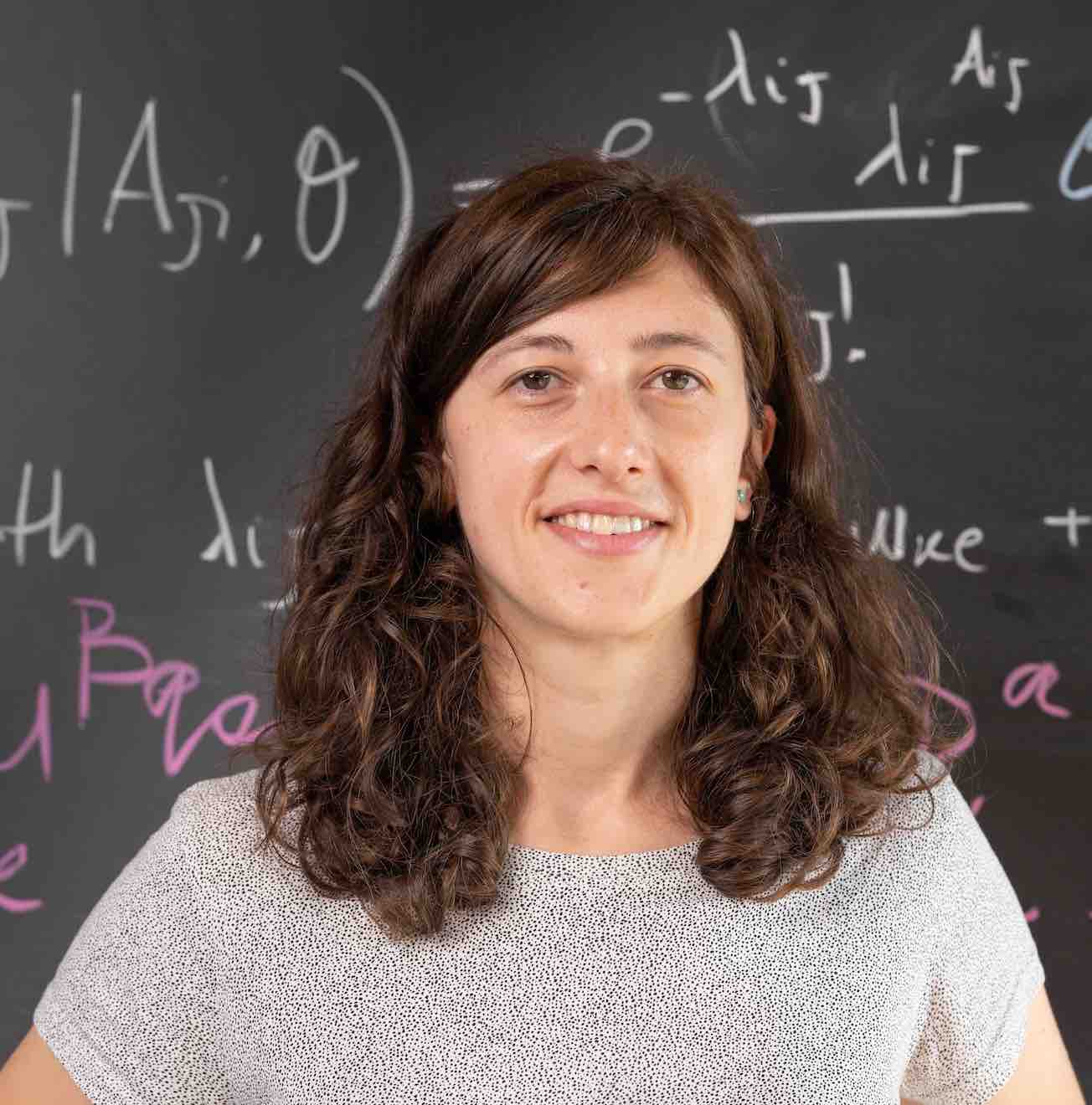
Caterina De Bacco is a Cyber Valley independent Group Leader at the Max Planck Institute for Intelligent Systems, Tuebingen, Germany. Prior to this, she spent sometime at the Santa Fe Institute, New Mexico and at the Data Science Institute at Columbia University for her postdoc positions. She obtained a Bachelor and Master degrees in Physics at Università of Padova, Italy and a PhD in Statistical Physics from Universitè Paris Sud, France. Her research focuses on studying interacting systems that can be modeled as networks from the perspective of statistical physics and probabilistic modeling. She investigates in particular the problems of performing inference tasks and routing optimization problems on networks. Some of her recent work focuses specifically on investigating systems with higher-order interactions using probabilistic generative models with latent variables. She focuses in particular on the challenge of developing analytically principled models that are also computationally efficient. Several algorithmic implementations of the hypergraph models developed together with her collaborators are available open source, including the library Hypergraphx (HGX).
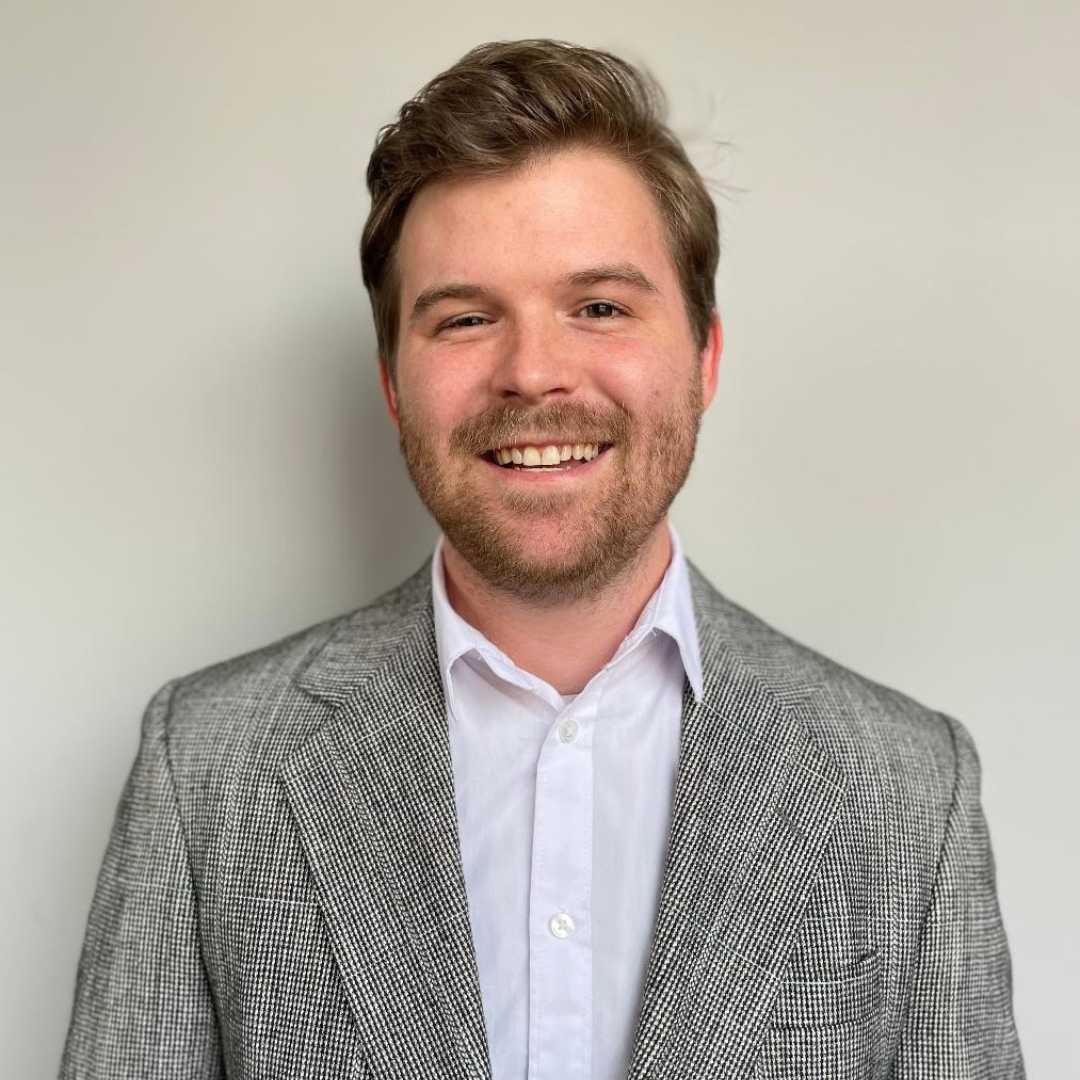
Audun is a data scientist at Pacific Northwest National Laboratory (PNNL). He received his PhD in Mechanical Engineering from Michigan State University in 2022 with a focus on data analysis methods applied to dynamical systems. His areas of research include topological data analysis, dynamical systems theory, time series analysis, (hyper)network science, and machine learning. His current work is focused on modeling and studying dynamical hypergraphs using tools ranging from graph and hypergraph theory, topological data analysis, and neural networks. He is also a contributor to PNNL’s open source HyperNetX library and works on bringing hypergraph analysis tools to this library.
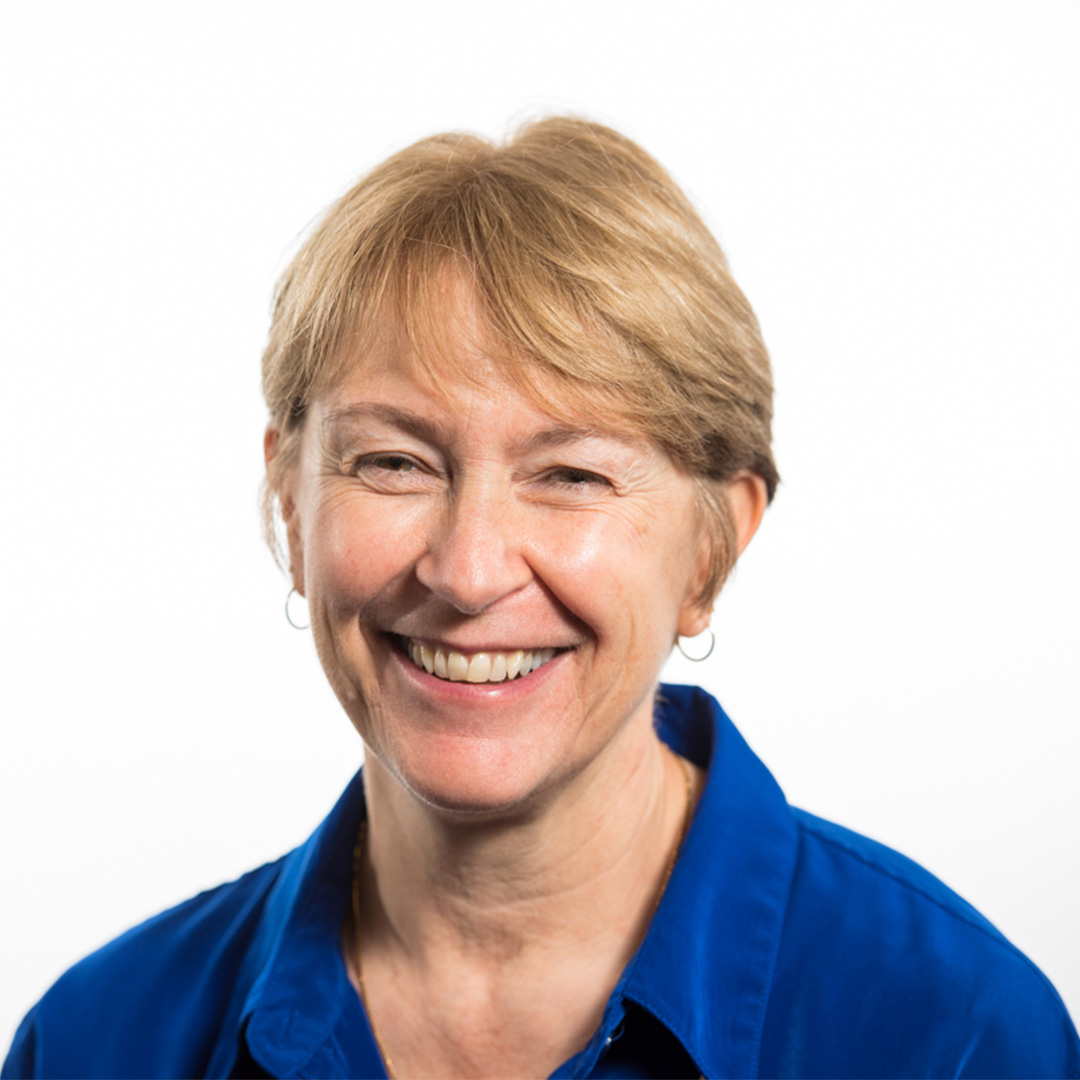
Brenda Praggastis, is a data scientist and mathematician at Pacific Northwest National Laboratory (PNNL). She received her BS and MA in Mathematics at SUNY, Stony Brook in 1987 and PhD in mathematics from the University of Washington in 1991 with a specialization in dynamical systems represented as shifts of finite type. She has been at PNNL since 2015. Her work has focused on knowledge graphs, applied topology, sheaf theory, deep learning and explainable AI. She led the development and the 2019 release of PNNL's open source library, HyperNetX (HNX), a Python library for the analysis and visualization of data modeled as hypergraphs. She currently leads the HNX team supporting numerous projects and the public face of HNX on Github. Since 2020 she has served as principal investigator for Deep Data Profiler: A Platform and Methodology for the Analysis and Interpretation of Neural Networks (DDP). Originally funded by PNNL's Mathematics for Artificial Reasoning in Science (MARS) initiative, DDP is in its fourth year. Both its team and scope have expanded to embrace the cutting edge of mechanistic interpretability research. As part of this work, she led the development and release of a second open source library, DeepDataProfiler (DDP), a Python library that models the decision process of a model data pairing as graph-like structures. When not doing data science, she enjoys traveling with her husband and their giant puppies in their truck camper, backpacking, river running, knitting, drawing, and being a grandmother.
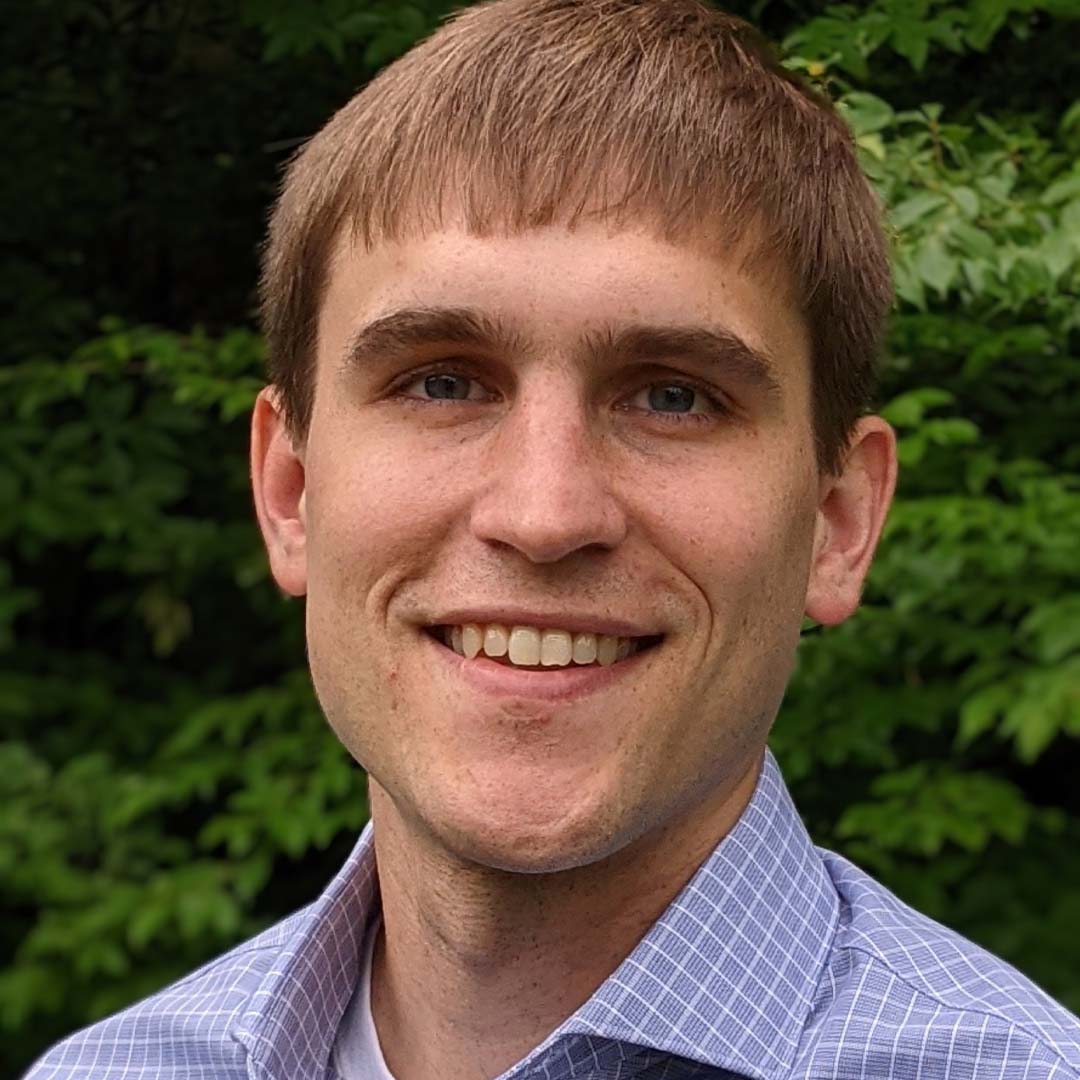
Nate Veldt is an assistant professor in the Department of Computer Science and Engineering at Texas A&M University. He is also an affiliated member of the Texas A&M Institute of Data Science. He obtained a bachelor's degree in mathematics from Wheaton College and a PhD in mathematics with a concentration in computational science from Purdue University in 2019. His research focuses on discrete algorithms and optimization for data science and network analysis. Much of his recent work focuses specifically on combinatorial algorithms for hypergraph analysis and their applications, including research on hypergraph partitioning, sparsification, and hypergraph-based clustering and semi-supervised learning.
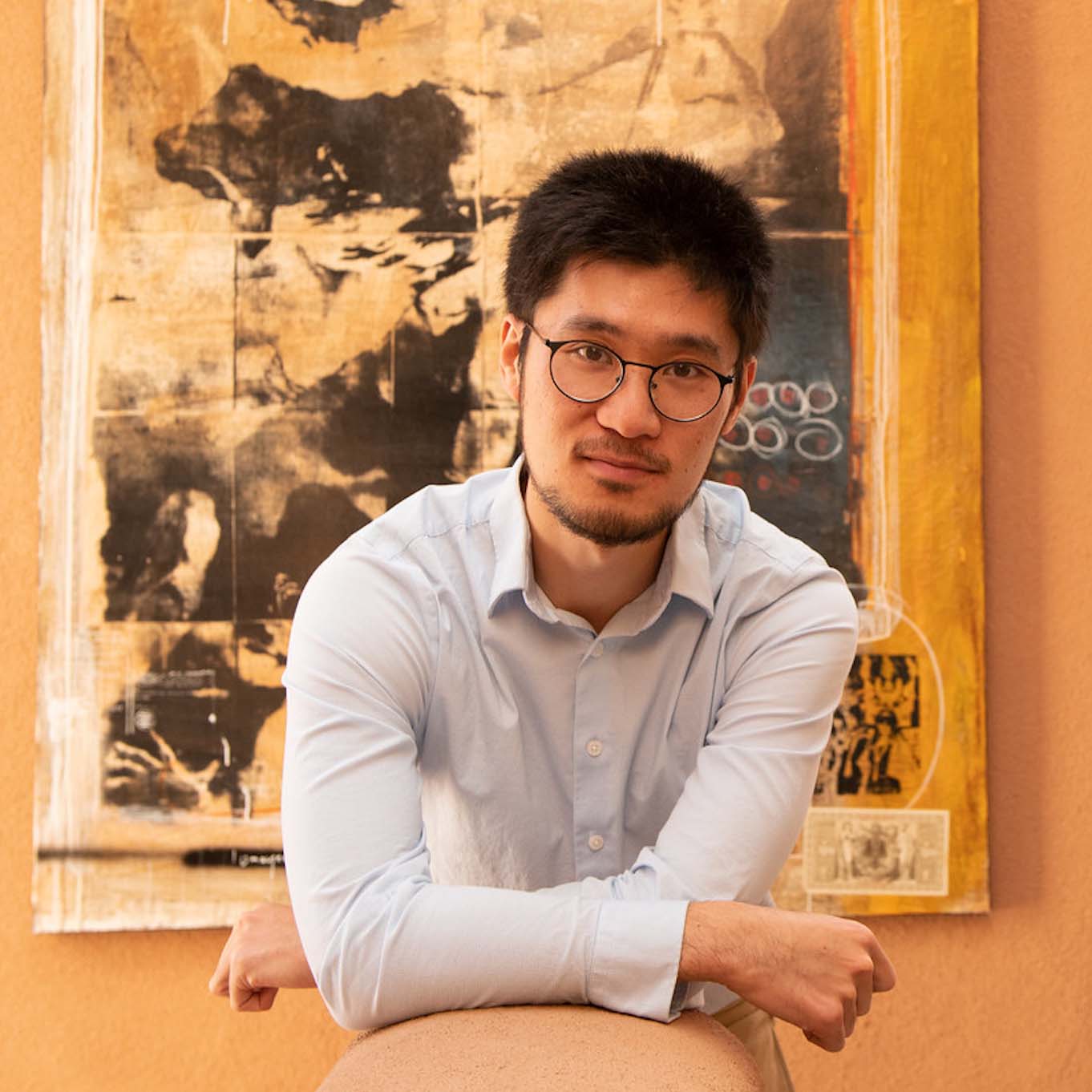
Yuanzhao is an Omidyar Fellow at the Santa Fe Institute. Before that, he was a Schmidt Science Fellow at Cornell working with Steven Strogatz. He got his Ph.D. in Physics from Northwestern in 2020, advised by Adilson Motter. His interest lies at the interface of networks and nonlinear dynamics. He draws techniques from dynamical systems, graph theory, and machine learning to understand the behavior of complex systems that are often nonlinear and high-dimensional. Some topics he worked on recently include synchronization on hypergraphs and simplicial complexes, basins of attraction in rugged landscapes, and data-driven modeling of dynamical systems.
Schedule
If the PDF links do not open, please try using another browser.